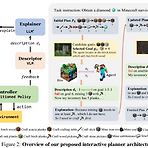
작성자 : 한양대학원 인공지능융합학과 유승환 박사과정 (CAI LAB)Keywords : Open-World, Interactive Planning, Multi-task Agents, Large Language Models, Minecraft논문 링크 : https://arxiv.org/abs/2302.01560 Describe, Explain, Plan and Select: Interactive Planning with Large Language Models Enables Open-World Multi-Task AgentsWe investigate the challenge of task planning for multi-task embodied agents in open-world environment..
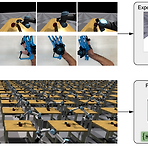
작성자 : 한양대학원 인공지능융합학과 유승환 박사과정 (CAI LAB) Keywords : Dexterous manipulation, Learning from human demonstrations, Reinforcement Learning 논문 링크 : https://ieeexplore.ieee.org/document/10000161 Accelerating Interactive Human-like Manipulation Learning with GPU-based Simulation and High-quality Demonstrations Dexterous manipulation with anthropomorphic robot hands remains a challenging problem in robot..
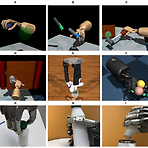
작성자 : 한양대학원 인공지능융합학과 유승환 박사과정 (CAI LAB) Keywords : Dexterous manipulation, Learning from human demonstrations, Reinforcement Learning 논문 링크 : https://link.springer.com/chapter/10.1007/978-3-031-19842-7_33 홈페이지 링크 : https://yzqin.github.io/dexmv/ DexMV: Imitation Learning for Dexterous Manipulation from Human Videos yzqin.github.io 영상 링크 : https://www.youtube.com/watch?v=scN4-KPhJe8 깃헙 링크 : https..
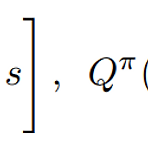
작성자 : 한양대학원 인공지능융합학과 유승환 박사과정 (CAI LAB) Keywords : Reinforcement Learning, Skill Extraction, Human Preferences 논문 링크 : https://proceedings.mlr.press/v164/wang22g.html Skill Preferences: Learning to Extract and Execute Robotic Skills from Human Feedback A promising approach to solving challenging long-horizon tasks has been to extract behavior priors (skills) by fitting generative models to large..
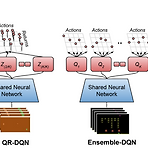
작성자 : 한양대학원 인공지능융합학과 유승환 박사과정 (CAI LAB) 논문 링크 : https://proceedings.mlr.press/v119/agarwal20c.html An Optimistic Perspective on Offline Reinforcement Learning Off-policy reinforcement learning (RL) using a fixed offline dataset of logged interactions is an important consideration in real world applications. This paper studies offline RL using the DQN rep... proceedings.mlr.press 홈페이지 링크 : https:..
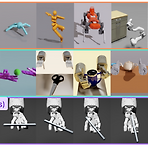
작성자 : 한양대학원 인공지능융합학과 유승환 박사과정 (CAI LAB) 논문 링크 : https://arxiv.org/abs/2310.12931 Eureka: Human-Level Reward Design via Coding Large Language Models Large Language Models (LLMs) have excelled as high-level semantic planners for sequential decision-making tasks. However, harnessing them to learn complex low-level manipulation tasks, such as dexterous pen spinning, remains an open problem. We bridg..
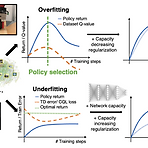
작성자 : 한양대학원 인공지능융합학과 유승환 박사과정 (CAI LAB) 논문 링크 : https://proceedings.mlr.press/v164/kumar22a.html A Workflow for Offline Model-Free Robotic Reinforcement Learning Offline reinforcement learning (RL) enables learning control policies by utilizing only prior experience, without any online interaction. This can allow robots to acquire generalizable skills from ... proceedings.mlr.press 홈페이지 링크 : Off..
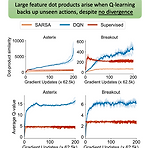
작성자 : 한양대학원 인공지능융합학과 유승환 박사과정 (CAI LAB) 안녕하세요!! 정말 오랜만의 블로그 포스팅입니다 ㅎㅎ 요새는 Offline Reinforcement Learning 분야를 연구하고 있습니다! 그런데 요녀석이 학습이 생각보다 잘 안돼더라고요 ㅜㅜ 저와 비슷한 고민을 하는 분들을 위해 오프라인 강화학습을 위한 팁! 논문을 가져왔습니다 ㅎㅎ 재밌게 읽어봐주세요~! 논문 링크 : https://openreview.net/forum?id=POvMvLi91f DR3: Value-Based Deep Reinforcement Learning Requires Explicit... Despite overparameterization, deep networks trained via supervised..